Demand sensing is a modern approach to forecasting in retail that uses real-time data and advanced analytics to predict customer demand more accurately. Unlike traditional demand planning, which relies heavily on historical sales patterns, demand sensing integrates up-to-date signals such as POS data, weather patterns, social media trends, and economic conditions. This enables retailers to create agile, short-term retail demand forecasts that better reflect current market realities.
Traditional methods assume the future will mirror the past, but in today’s dynamic retail environment, that’s no longer sustainable. Demand sensing is becoming increasingly critical as companies face rapidly changing consumer preferences, complex global supply chains, and frequent market disruptions. Whether it’s a viral trend, an economic shift, or a natural disaster, real-time forecasting helps retailers respond fast by avoiding stockouts, reducing excess inventory, and improving customer satisfaction.
With demand constantly in flux, the ability to sense and adapt quickly is a competitive advantage. By shifting from static planning to real-time responsiveness, demand sensing is redefining how retailers forecast, plan, and win in a fast-changing world.
The importance of demand sensing in modern retail
Picture this: a trending influencer promotes a product, and demand spikes overnight. Retailers using traditional methods miss the opportunity. But with demand sensing in retail, brands can tap into real-time insights and adjust instantly. Demand sensing empowers retailers to better understand and anticipate customer behavior, using analytics to not only improve demand forecast accuracy, but also optimize pricing, promotions, and product availability. By predicting market trends and aligning supply with real-time demand, businesses can stay ahead of the competition.
Modern tools combine both online and offline data to detect trends and forecast variances quickly. This leads to faster, smarter decisions that drive better performance across critical KPIs.
For instance, supply chain KPIs benefit through improved perfect order rates, reduced emergency shipments, and lower inventory carrying costs. On the financial side, demand sensing helps increase profit margins, capture additional revenue during demand surges, and shorten cash-to-cash cycle times by reducing excess stock.
In short, demand sensing in retail isn’t just about efficiency—it’s about gaining a competitive edge through agility, accuracy, and insight-led decision-making.
How demand sensing works in retail
Imagine a sudden spike in demand for umbrellas due to an unexpected weather forecast. Traditional forecasting models wouldn’t catch it until it’s too late, but, demand sensing technology would. That’s because demand sensing relies on real-time data, advanced analytics, and machine learning to enhance short-term forecasting accuracy and help retailers react instantly to shifting demand.
When implementing demand sensing into your supply chain, consider the following five key components and two best practices. Together, these elements power the full potential of demand sensing tech and enable more responsive, accurate retail forecasting.
1. Data Collection
The foundation of demand sensing is high-quality, real-time data. Retailers must collect data from internal sources like point-of-sale (POS) systems, e-commerce platforms, inventory management tools, and loyalty programs. To enhance accuracy, external signals such as weather forecasts, economic trends, and social media sentiment are also gathered. The goal is to build a holistic picture of consumer behavior, market drivers, and demand fluctuations.
2. Data Integration
Next, the collected data must be centralized and made ready for analysis. This is done through the ETL (Extract, Transform, Load) process:
- Extraction: Pulls data from various systems and sources, both structured and unstructured.
- Transformation: Cleans and standardizes data by removing errors, duplicates, and formatting inconsistencies.
- Loading: Pushes the refined data into a centralized platform or demand sensing software for analysis.
Be cautious, as there are challenges in integrating external data, such as inconsistent formats or missing values. However, these can be addressed through data wrangling, API integration, and strong data governance policies.
3. Data Analysis
Once integrated, the data is analyzed using both statistical and machine learning techniques. Retailers may apply regression models to understand price sensitivity or seasonal effects. Meanwhile, machine learning algorithms—like decision trees and neural networks—identify hidden patterns, anomalies, and relationships between variables that would otherwise be missed. This step is critical for generating actionable insights in near real-time.
4. Data Modeling
Based on the analysis, predictive models are developed to forecast demand in the short term (typically 0–14 days). These models ingest real-time inputs—such as marketing promotions, weather changes, or social buzz—and are updated continuously. The flexibility and adaptability of these models are what give demand sensing software its edge over traditional forecasting tools.
5. Data Optimization
Finally, predictive models inform and optimize business decisions across the supply chain. If a spike in demand is detected for a certain SKU, inventory can be reallocated, production schedules can be adjusted, and marketing efforts can be fine-tuned. This leads to reduced inventory waste, better service levels, and increased profitability.
By combining these five steps, demand sensing empowers retailers to act with speed, accuracy, and agility. It turns complex, fast-moving data into clear insights that drive better outcomes across merchandising, logistics, and customer satisfaction.
Best Practices:
- Data Reconciliation: Align demand sensing insights with existing planning processes to avoid conflicts and ensure continuity.
- Continuous Improvement: Treat demand sensing as a learning system—refine models as new data becomes available for greater accuracy over time.
Benefits of implementing demand sensing in retail
Adopting demand sensing in retail offers significant competitive advantages, especially in today’s fast-moving, consumer-driven market. By leveraging real-time data and advanced analytics, retailers can enhance their retail demand forecast accuracy and make faster, smarter decisions across the supply chain.
One of the biggest advantages is improved forecast precision. Traditional forecasting methods often rely on outdated, historical data, which can’t keep up with sudden market changes. Demand sensing, on the other hand, uses real-time inputs—such as POS data, online trends, weather changes, and promotions—to fine-tune short-term forecasts. This enables retailers to better match supply with actual demand, reducing stockouts and excess inventory.
Another key benefit is greater agility. With demand sensing, retailers can respond quickly to demand shifts, seasonal trends, and external disruptions like economic changes or viral product trends. This flexibility helps avoid costly markdowns, improves inventory turnover, and increases customer satisfaction.
Additionally, demand sensing in retail supports stronger collaboration across departments—aligning merchandising, planning, and supply chain teams on a shared, data-driven view of demand. It also enables smarter allocation of inventory across channels, ensuring the right products are available in the right place at the right time.
Ultimately, enhancing your retail demand forecast with demand sensing leads to reduced operational costs, improved margins, and a more resilient supply chain. Retailers who adopt these techniques aren’t just adapting, they’re thriving in an environment where speed and accuracy are everything.
Demand sensing vs traditional demand forecasting
Feature | Traditional forecasting | Demand sensing |
---|---|---|
Data inputs | Historical sales only | Real-time & historical data |
Update frequency | Weekly or monthly | Daily or hourly |
Forecast horizon | Long-term | Short-term |
Responsiveness | Low | High |
Accuracy | Moderate | Significantly higher |
Traditional forecasting in retail relies on historical data and statistical models, often updated monthly or quarterly. While this approach supports long-term planning, it lacks the agility needed to respond to today’s fast-changing market dynamics. Demand sensing, by contrast, is designed for real-time responsiveness—using current data from POS systems, online behavior, weather trends, and even social media to create highly accurate, short-term forecasts.
In terms of demand forecast accuracy, demand sensing significantly outperforms traditional methods. It can reduce forecast errors by up to 40%, giving retailers a sharper understanding of what customers want, when, and where. This means fewer stockouts, lower inventory costs, and better alignment between supply and demand.
More importantly, demand sensing enables rapid decision-making. In times of disruption—such as during the COVID-19 pandemic—retailers relying solely on historical forecasting struggled to keep up with shifting consumer behavior. Meanwhile, companies leveraging demand sensing adjusted production and inventory levels in real time, staying in sync with unexpected demand surges or declines.
By incorporating diverse data sources and leveraging machine learning, demand sensing provides a more comprehensive, real-time view of demand. It empowers retailers to act quickly, optimize inventory, and execute pricing and promotional strategies more effectively.
In short, demand sensing doesn’t just improve demand forecast accuracy—it transforms forecasting into a proactive, dynamic process that supports greater resilience and smarter retail operations.
Key Takeaways
In today’s fast-moving retail environment, the ability to sense and respond to demand shifts in real time is no longer optional—it’s a competitive necessity. Demand sensing bridges the gap between static, long-term forecasting and the dynamic realities of modern consumer behavior. By leveraging real-time data, advanced analytics, and machine learning, retailers can improve forecast accuracy, reduce inventory risk, and deliver better customer experiences.
Whether you’re managing seasonal surges, reacting to viral trends, or navigating supply chain disruptions, demand sensing provides the agility and insight needed to stay ahead. Retailers that embrace this shift are not only more responsive—they’re more resilient, more efficient, and ultimately, more profitable.
About the author
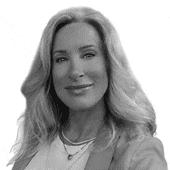
Karen McNaughton
Karen is the Vice President of Global Marketing at Algo, where she leads strategies to enhance brand awareness and demand generation for the company’s supply chain intelligence platform. With over twenty years of experience in senior marketing roles at various SaaS technology organizations, Karen brings extensive expertise in leading global marketing teams and executing go-to-market strategies.